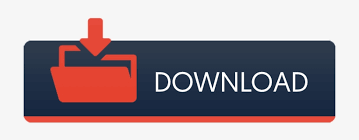
The first example compares 14 antimanic drugs for acute mania. To enhance interpretation of all presented graphical and numerical summaries we used three worked examples of NMAs.
#STATA COLORS FULL#
We provide the script files for the full analysis in the Appendix S1.ġ Examples of network meta-analyses and STATA routines Finally, Section 6 focuses on suggestions for graphical and numerical presentation of the results. In Section 5 we describe graphical and numerical ways to display important assumptions of the joint analysis.
#STATA COLORS HOW TO#
Section 4 briefly summarizes how to fit a NMA model using mvmeta in STATA. In Section 3 we discuss graphs that enhance understanding of the dataset and facilitate the visualization of the evidence with respect to its characteristics. The structure of the rest of the paper follows a typical analysis of a network of randomized controlled trials. We first present in Section 2 three working examples that will be used to present the developed STATA routines. A thorough review of statistical methodology for NMA has been described previously. Emphasis is placed on practical issues of applying and presenting NMA. We created STATA routines that extend existing graphical tools used in pairwise meta-analysis and we also developed new tools specifically for NMA. With this important development as our starting point, we introduce a suite of STATA routines to evaluate the assumptions and graphically present NMA results.
#STATA COLORS UPDATE#
As the number of competing treatments included in a networks of interventions increases, the need for a concise and informative presentation of results becomes more important.Ī recent update in the multivariate meta-analysis routine in STATA ( mvmeta command) makes NMA possible within one of the most widely used software for meta-analysis, and we expect that this will popularize the method. Finally, NMA results are not easy to interpret and do not always facilitate decision-making. Graphical tools may help investigators to spot parts of the evidence network that appear inconsistent, or to inform judgments about the plausibility of consistency. Valid results from NMA depend on the evidence network being internally consistent: direct and various sources of indirect evidence should be in agreement. Funnel plots to identify the presence of small-study effects provide further challenges in a NMA context because observed effect sizes refer to different treatment comparisons. Heterogeneity and its impact on summary estimates are also difficult to display graphically – or even numerically – in an understandable way. For example, forest plots facilitate the inspection of the evidence base and its characteristics but may be less informative when several comparisons are present. Many of these tools are difficult to apply to NMA without modifications. Various plots have been suggested for summarizing the evidence from studies on the relative effectiveness or acceptability of two interventions. Graphical tools can provide comprehensive and easily understandable ways to present results of statistical analyses, particularly when a large amount of data is involved. The lack of a user-friendly implementation framework with tools to evaluate the assumptions of the analysis and present the results has contributed to this criticism. It has been criticized for its complexity, for involving assumptions that are difficult to evaluate and for producing outputs that cannot be easily understood and interpreted by clinicians. However, NMA remains, to a large degree, a privilege for researchers with advanced computational and statistical knowledge. The advantages of NMA have made it an increasingly popular method in comparative effectiveness research. The assumption of consistency (agreement between direct and indirect sources of evidence) underlies the methodology and if it holds, NMA can give valuable information to patients, practitioners and decision makers. The integration of direct evidence (from studies directly comparing interventions) with indirect evidence (information about two treatments derived via a common comparator) increases the precision in the estimates and produces a relative ranking of all treatments for the studied outcome. Network meta-analysis (NMA) synthesizes data from a network of trials about more than two competing healthcare interventions.
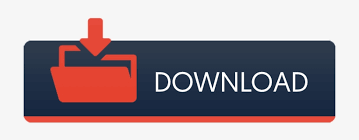